
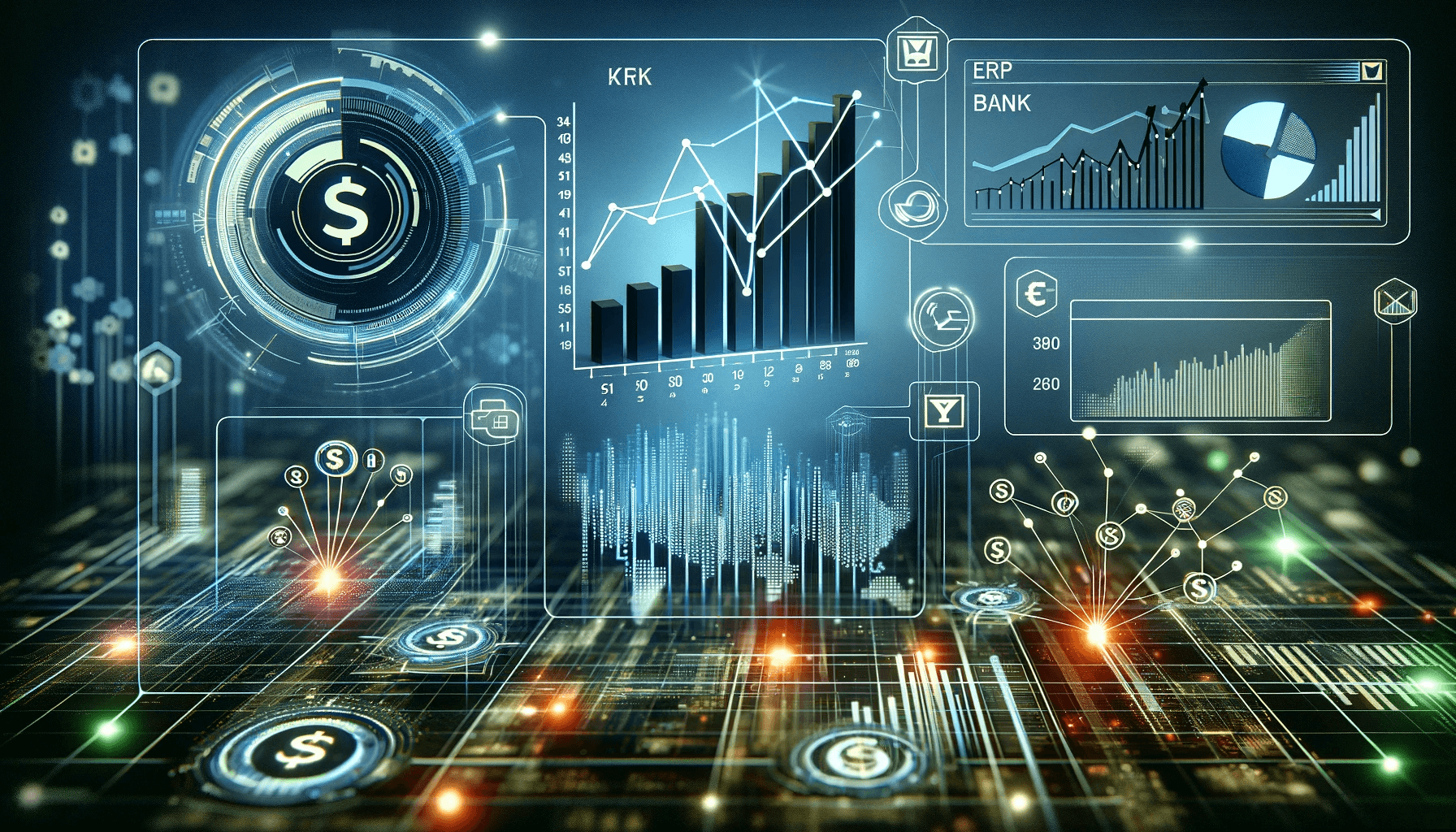
Cash forecasting should be viewed as a central pillar of intelligent treasury management. While the capability to connect to multiple banks and display current balances and transactions has become table stakes in the TMS (treasury management system) market, the ability to accurately project cash positions over the short, medium, and long term is still an area of great opportunity and promise. Some TMS platforms, including Balance, provide robust and flexible forecasting tools that evoke an evolutionary leap compared to spreadsheets with complex formulas spread across multiple tabs. These tools introduce automation that allows users of all backgrounds to quickly and easily build robust forecasts using historical transaction data, ERP data, and simple tools for building dynamic recurring patterns from scratch.
Historical transactions data is oftentimes an optimal starting point for a new forecast. An existing organization already has established financial patterns. For example, most organizations have somewhat predictable monthly revenues, which are sometimes subject to seasonal fluctuations. A real estate business will collect rent each month from tenants, a software business will collect licensing revenues, and so on. Most organizations also have a category of predictable monthly expenses, such as payroll, rent, and insurance. By looking back at one to two years of historical patterns, we can calculate a monthly baseline of inflows and outflows by category and we can also observe seasonality and growth.
ERP integration adds another layer to the historical transaction patterns that can be deduced from bank data. On the most basic level, the ERP system contains AR and AP data, which is fairly universal across industries. On a more granular level, the cash forecast can incorporate long-term contracts, such as lease agreement in a real estate management context. If the ERP system contains a 5-year lease that grows at 5% per year for the first 2 years and 3% for the subsequent 3 years, an intelligent forecast can incorporate those terms. Furthermore, the forecast can predict payment date based on historical precedent. Some customers will pay on the first of the month, while others may wait until the 5th, or later.
Finally, a forecast must facilitate the creation of cash movements that are not yet reflected in the bank history or the ERP. Traditionally, such forecasts have been built in spreadsheets, with embedded formulas for growth over time. While this is a practical approach to pro forma modeling, the spreadsheet cannot easily analyze historical transactions to identify patterns. Similarly, incorporating ERP data is often a complex manual process. A robust cash forecast allows the user to build a recurring inflow or outflow from scratch and also incorporates patterns and ERP data.
The capability to quickly generate an accurate forecast based on bank and ERP integration not only saves time, but also enhances accuracy. The modeler no longer must rely on memory and re-keying to build out a complex and non-standardized model that becomes stale as soon as a bank account balance changes. Balance combines data that was previously siloed while increasing the reliability and freshness of a cash forecast.